Unlocking Potential: Healthcare Datasets for Machine Learning
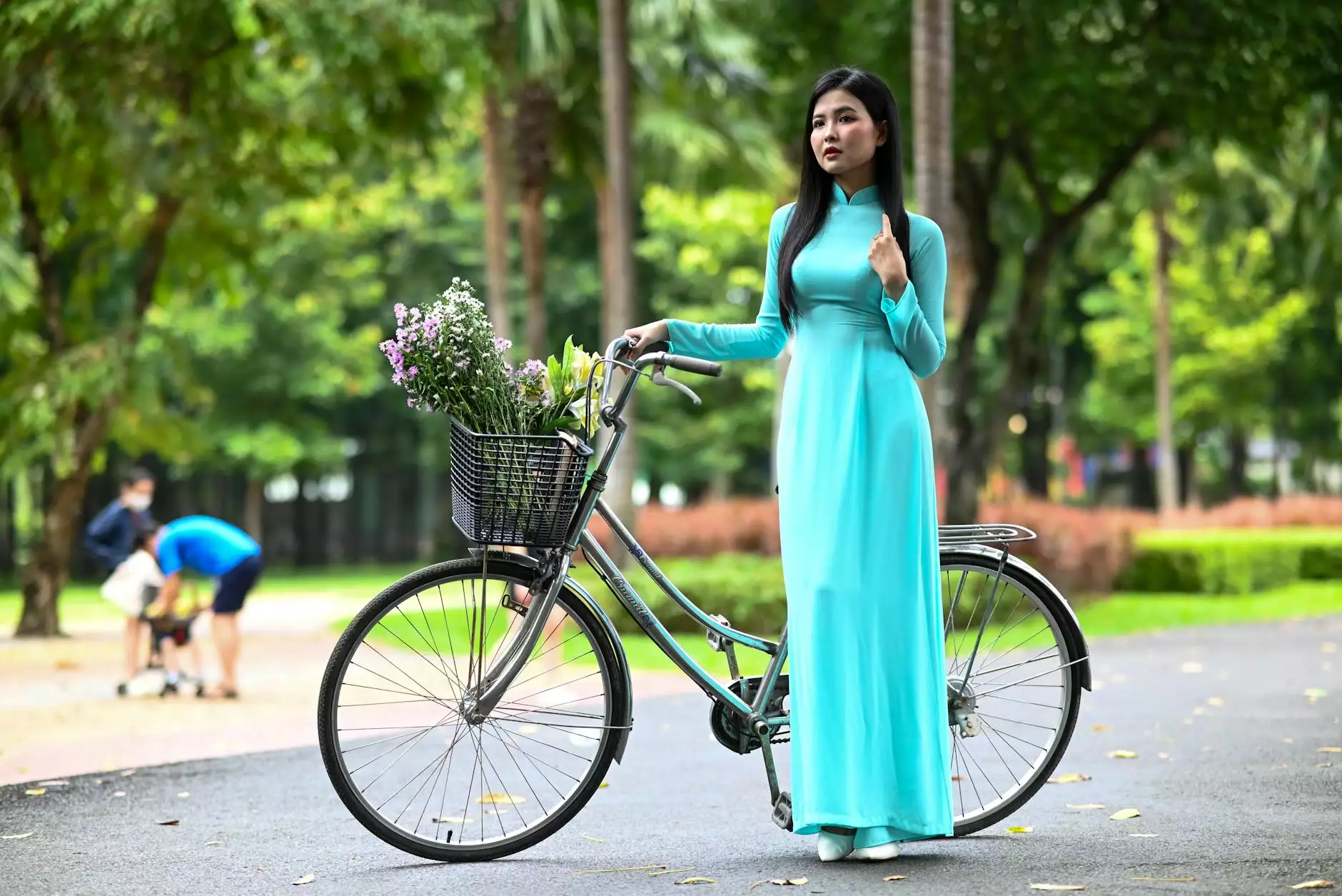
In today's rapidly evolving digital world, the intersection of healthcare and technology has paved the way for unprecedented advancements in patient care, operational efficiency, and research capabilities. Among the most promising innovations in this realm is the application of machine learning (ML) within the healthcare sector, driven largely by the utilization of extensive and rich healthcare datasets for machine learning. This article delves into the importance of these datasets, their applications, benefits, challenges, and the future landscape they are shaping in healthcare.
Understanding Healthcare Datasets
Healthcare datasets encompass a wide variety of data types, formats, and sources, including:
- Electronic Health Records (EHRs): Comprehensive patient health records that include demographics, medical history, medications, lab results, and treatment plans.
- Medical Imaging Data: Datasets derived from various imaging technologies such as MRI, CT scans, and X-rays.
- Clinical Trials Data: Data generated from clinical studies that evaluate the efficacy of new treatments and interventions.
- Patient Surveys and Feedback: Information gathered directly from patients regarding their experiences and satisfaction with treatments.
- Wearable Device Data: Metrics collected from fitness trackers and medical devices that monitor vital signs and physical activity.
Why Utilize Healthcare Datasets for Machine Learning?
The integration of machine learning with healthcare datasets offers a multitude of advantages:
1. Enhanced Diagnostic Accuracy
Machine learning algorithms can analyze vast amounts of data to identify patterns that may not be immediately visible to human practitioners. For instance, ML can improve the diagnostic accuracy of diseases such as cancer by analyzing imaging data against a backdrop of historical patient information.
2. Predictive Analytics
By leveraging healthcare datasets for machine learning, providers can utilize predictive models to forecast disease outbreaks, patient admissions, and individual patient risk factors. This proactive approach can lead to improved health outcomes and reduced costs.
3. Personalized Medicine
Machine learning enhances the ability to personalize treatment plans based on individual patient data. By analyzing genetic information alongside traditional health records, healthcare providers can develop targeted therapies tailored specifically to the patient’s needs.
4. Operational Efficiency
Hospitals and clinics can employ ML algorithms to optimize resource allocation, streamline patient flow, and manage supply chains more effectively. This results in reduced waiting times and improved patient satisfaction.
Challenges in Using Healthcare Datasets for Machine Learning
While the benefits are substantial, leveraging healthcare datasets for machine learning is not without its challenges:
1. Data Quality and Standardization
The quality of healthcare data can vary significantly. Inconsistencies across systems, formats, and terminologies can hinder the effectiveness of machine learning models. Establishing standardized data practices is essential for ensuring data reliability.
2. Privacy and Security Concerns
Healthcare data is sensitive, and the need to protect patient information is paramount. Ensuring compliance with regulations such as HIPAA in the U.S. is crucial for any organization wanting to utilize healthcare datasets for ML.
3. Interpretability of Models
Machine learning models, especially those based on deep learning techniques, can be seen as "black boxes". Understanding how these models reach their conclusions is vital in healthcare, where decisions can significantly affect patient outcomes.
4. Integration with Existing Systems
Integrating machine learning solutions into existing healthcare infrastructure can be complex. Stakeholders need to ensure seamless data flow between systems.
Applications of Machine Learning in Healthcare
Healthcare institutions worldwide are beginning to recognize the potential of healthcare datasets for machine learning. Here are some of the significant applications:
1. Clinical Decision Support Systems (CDSS)
Machine learning models are increasingly being implemented in CDSS to aid healthcare professionals in making informed decisions. These systems can analyze patient data and suggest treatment plans or alert providers about potential drug interactions.
2. Medical Imaging Analytics
Algorithms trained on medical imaging datasets can identify anomalies in scans that might be missed by human eyes. For instance, AI systems can detect early signs of diabetic retinopathy in retinal images, leading to timely interventions.
3. Drug Discovery and Development
Machine learning is transforming the pharmaceutical industry by optimizing drug discovery processes. Companies can analyze vast datasets to identify potential drug candidates and predict their efficacy and safety profiles much earlier in the development process.
4. Remote Patient Monitoring
Wearable devices collect continuous health data, allowing machine learning algorithms to monitor patient health remotely. This is especially useful for managing chronic diseases, where patient adherence to treatment plans is critical.
5. Natural Language Processing (NLP)
NLP applications in healthcare utilize machine learning to analyze unstructured data in clinical notes and patient feedback. This helps extract valuable insights to improve care delivery and patient engagement.
The Future of Healthcare Datasets and Machine Learning
As the healthcare landscape evolves, the future of healthcare datasets and machine learning looks promising:
1. Increased Interoperability
Efforts are ongoing to enhance interoperability between different healthcare data systems. This will allow for more comprehensive datasets, which can improve the performance of machine learning models.
2. Greater Focus on Patient-Centric Care
With machine learning's ability to analyze patient-reported outcomes, healthcare will trend towards even more personalized and patient-centric approaches.
3. Enhanced Training and Resources for Healthcare Professionals
As more healthcare organizations adopt machine learning, there will be a corresponding need for training programs to equip healthcare professionals with the knowledge and tools to effectively utilize these technologies.
4. Ethical Considerations in AI
The discussion around ethical AI in healthcare will intensify. Ensuring that machine learning applications are used safely and responsibly while promoting equity in health outcomes will be paramount.
Conclusion
The integration of healthcare datasets for machine learning is transforming the healthcare industry by enhancing diagnostic accuracy, improving patient outcomes, and optimizing operational efficiency. While there are challenges to address, the potential benefits far outweigh them, making it an exciting area for innovation. As technology continues to evolve, we can expect further advancements that will enhance the quality of healthcare and redefine patient experiences.
At keymakr.com, we are dedicated to delivering high-quality services in our Home Services, Keys & Locksmiths niche. We understand that technology plays a significant role in enhancing our services and operations, much like machine learning is revolutionizing healthcare. Stay tuned for more insights as we explore the transformative power of technology across various sectors.